Next: Models
Up: Zmapqtl
Previous: Zmapqtl
  Contents
  Index
Composite interval mapping [ZengZeng1993,ZengZeng1994] combines
interval mapping with multiple regression. The statistical model is defined as
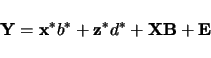 |
(3.5) |
where
is a vector of trait values
and
are the additive and dominance effects of the
putative QTL being tested
-
and
are indicator variable vectors
specifying the probabilities of an individual being in different genotypes for
the putative QTL constructed by flanking makers
is the vector of effects of other selected markers fitted in
the model
is the marker information matrix for those selected markers
is the error vector.
Estimates of the parameters are obtained by maximum likelihood through an ECM
(for Expectation/Conditional Maximization) algorithm [Meng and RubinMeng and
Rubin1993]. In each
E-step, the probability of an individual being in different genotypes of the
putative QTL is updated. In the CM-step, the estimation of parameters
and
is separated from that of
, and each group is estimated
conditional on the others. This procedure is implemented for numerical
consideration. As
and
are separated from
,
is unchanged in each iteration, and its costly
recalculation is avoided.
For an
population, the hypotheses for testing are
and
. This is performed through a likelihood ratio test procedure. In
addition, it is possible to test hypotheses on
and
individually. For a backcross data set, dominance cannot be estimated and
is dropped from Equation 3.5.
The trait will have a variance
. Under the null hypothesis
the sample variance of the residuals will be
. For a given
alternative model, say
the variance of the residuals would be
. With this in mind we can
calculate the proportion of variance explained by a QTL at the test site.
The quantity is usually called
and estimated by
An alternative estimate would use the total variance. Denote it by
is the proportion of the variance explained by the QTL conditioned on the
background markers and any explanatory variables.
is the proportion of the
total variance explained by the QTL and the the background markers and any explanatory
variables. Generally,
Next: Models
Up: Zmapqtl
Previous: Zmapqtl
  Contents
  Index
Christopher Basten
2002-03-27